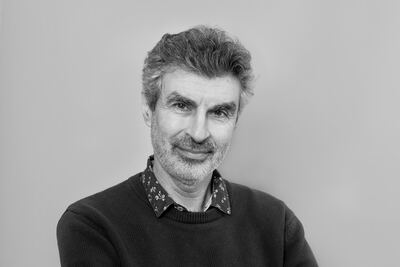
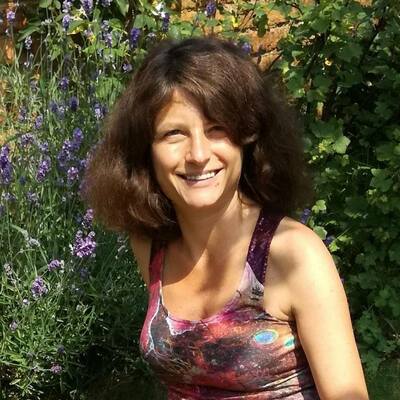
Sony believes that AI and Robotics technology can activate and augment human capabilities and abilities. In 1999, Sony introduced AIBO to the consumer market. This was a four-legged autonomous robot with pseudo instincts and emotions that could interact with people like pets. The responses of the non-verbal robot was interpreted by humans with some degrees of freedom and it was this freedom of the interpretation that contributed to the smoothness of the interactions.
Then in 2010, Sony developed a robot prototype called Rudy, which focused on more specifically physical interaction between humans and robots This feature was achieved by a special force-controlled actuator (VA) and a method called generalized inverse dynamics (GID) calculation, which enabled Rudy to physically interact with objects and humans in the real world safely. For example, it was able to assist a physically handicapped patient to control Rudy with a simple GUI to fetch a towel on a shelf. This was an example of augmenting human’s physical capability.
The VA and GID was then also applied for bilateral-controlled manipulator to augment the human hand’s skills and capability. Using micro-end effector equipped with dedicated force sensors, we achieved to augment and improve the accuracy of the force sensitivity and position of the human hand by 10 times..
Now as we consider the AI and Robotics in the field of Gastronomy, we are exploring the possibilities of augmenting the chef’s imagination and creativity for new recipe generation, and the Chef’s skills in the actual cooking process.
In this talk, I will explain the activation and augmentation of these various human abilities, and the behavior control architecture behind them.
Masahiro Fujita graduated from Waseda University in 1981 and received a Master’s degree (MSEE) from University of California Irvine in 1989. In 1981, he joined Sony Corporation and developed the entertainment robot AIBO, which is fully autonomous quadruped dog-like robot, and the small humanoid robot QRIO, which can dynamically walk, run, dance, sing, and talk as entertainment purpose. Afterwards, he studied a new approach to realize self-development intelligence focusing on embodied motion dynamics called Intelligence Dynamics, where AI system learns predictors and controllers using input and predicted input, so there is no labeled data necessary, and explore actions to goal state states set by flow theory based approach. While working on Intelligence Dynamics, he studied force control robotics for remote-operated robots with physical contact capability safely, and for bilateral manipulator augmenting human hand skills. In 2018, he started some new activities related to AI and Robotics such as AI Ethics and Gastronomy project. He led to release Sony Group AI Ethics Guidelines in 2018 and to establish Sony Group AI Ethics Committee in 2019, and he is the head of the Secretariate. He is now VP, and Senior Chief Research Researcher of Sony Group Corp., and Director of Sony AI Inc.
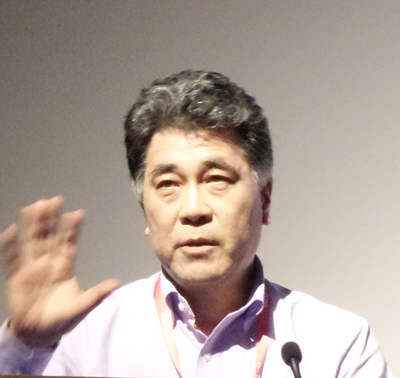
Dr. Sameena Shah is a Managing Director in the AI Research organization at J.P. Morgan. She is a highly accomplished technology leader with over 20 years of educational and industry experience in engineering, AI, and leading development teams that created top AI technologies in the world for financial, news, risk and legal businesses.
Previously, Sameena was Managing Director, Head of Data Science at S&P Global Ratings where she led the firm’s strategy and development for Augmented Intelligence. Prior to that, Sameena was Director of Research at Thomson Reuters, where along with her team, she built award winning AI systems that resulted in significant business growth and operational efficiencies. Sameena is also the Founder and CEO of Aylan, and has worked at Yahoo! Research, a NYC based hedge fund, an International hedge fund, and a global startup.
Sameena has a PhD in Distributed Machine Learning and a Masters in Computer Science from IIT Delhi. She is the winner of the top PhD in the country award, Cloudera top AI/ML application award, several best paper awards and recognitions. She has contributed more than 50 publications, and 30 patents.
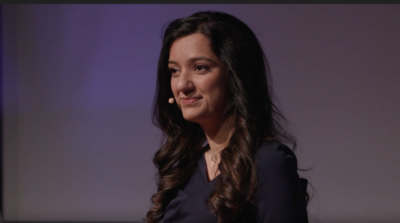
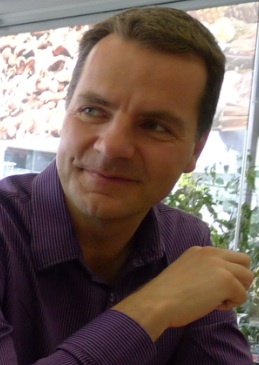
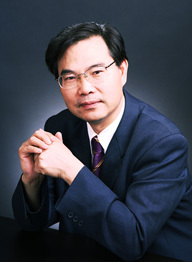